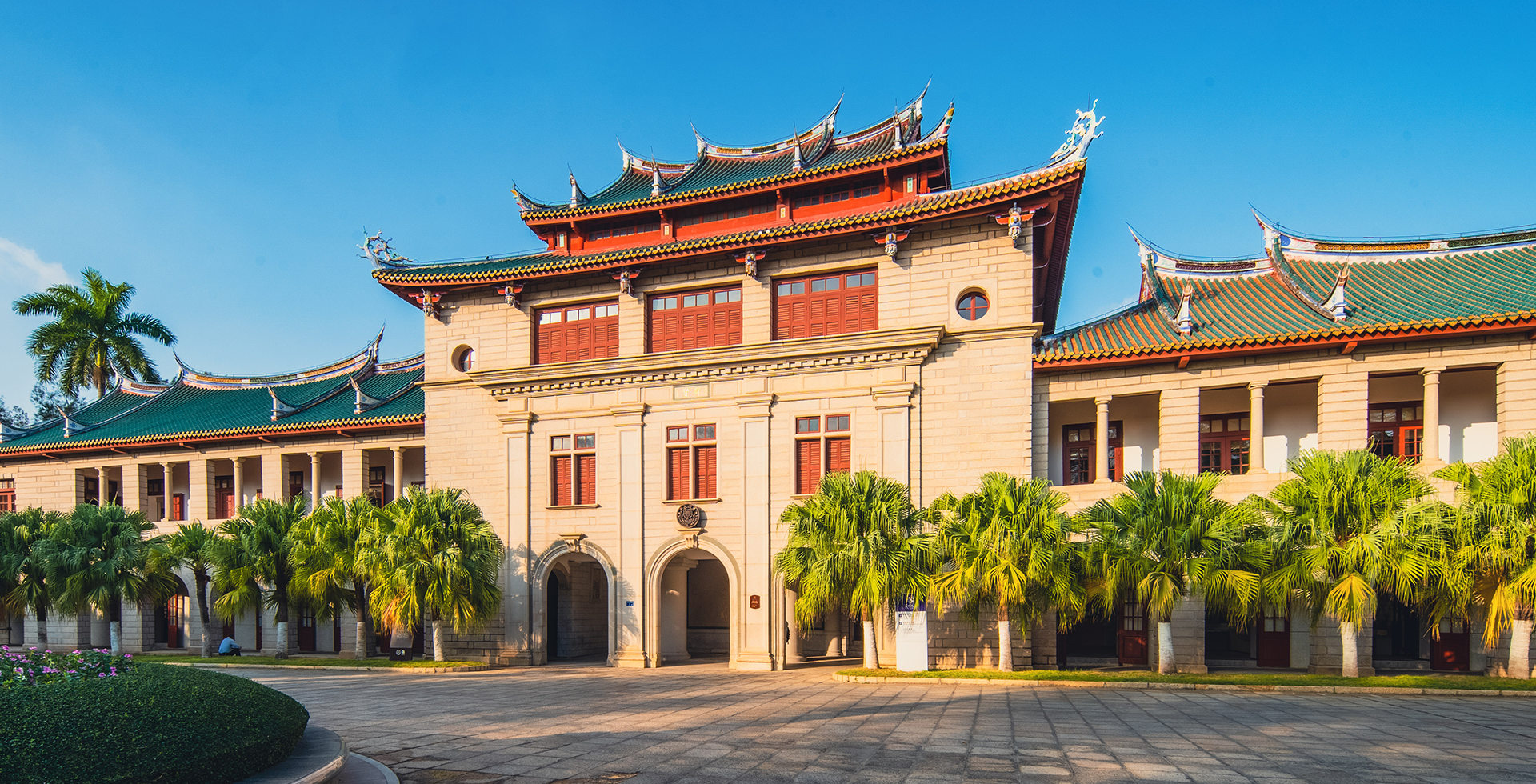
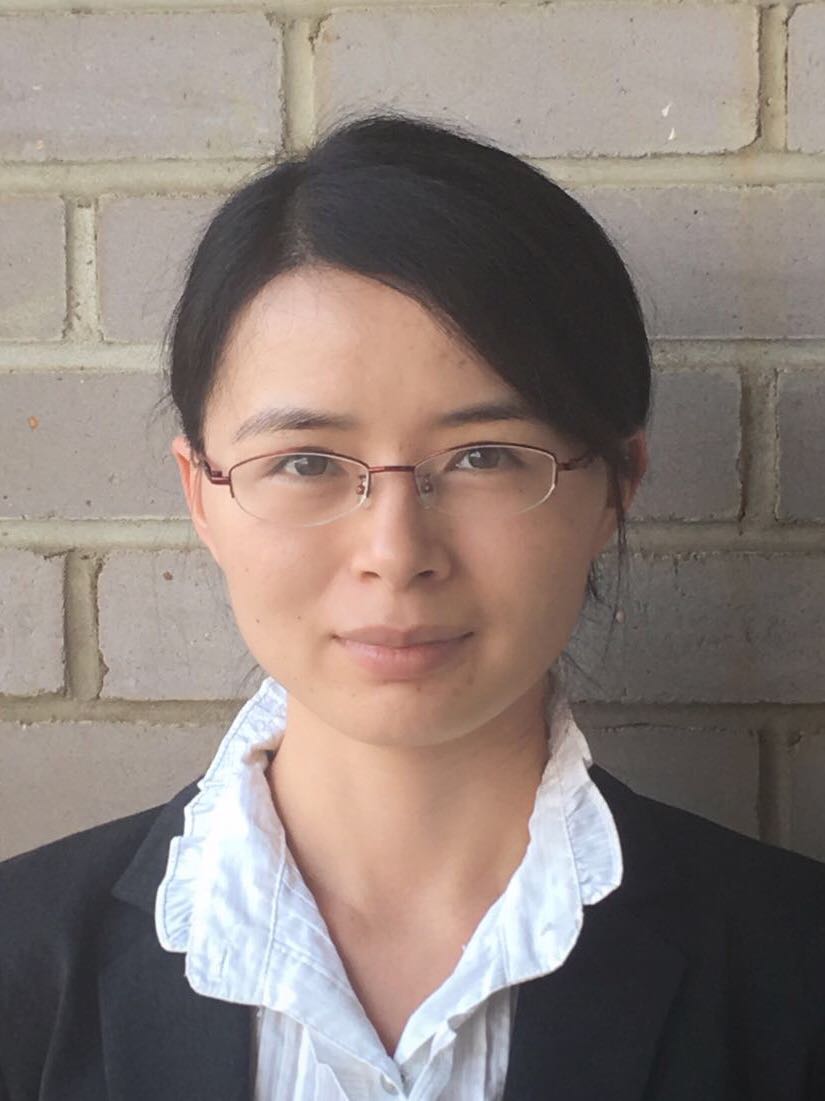
Published Papers
Efficient peer effects estimators with group effects (with Guido Kuersteiner and Ingmar Prucha), Journal of Econometrics 235, 2023, p. 2155–2194.
Working Papers
Differential Test Performance and Peer Effects (with Guido Kuersteiner and Ingmar Prucha)
We use variation of test scores measuring closely related skills to isolate peer effects. The intuition for our identification strategy is that the difference in closely related scores eliminates factors common to the performance in either test while retaining idiosyncratic test specific variation. Common factors include unobserved teacher and group effects as well as test invariant ability and factors relevant for peer group formation. Peer effects work through idiosyncratic shocks which have the interpretation of individual and test specific ability or effort. We use education production functions as well as restrictions on the information content of unobserved test taking ability to formalize our approach. An important implication of our identifying assumptions is that we do not need to rely on randomized group assignment. We show that our model restrictions are sufficient for the formulation of linear and quadratic moment conditions that identify the peer effects parameter of interest. We use Project STAR data to empirically measure peer effects in kindergarten through third grade classes. We find evidence of highly significant peer effects with magnitudes that are at the lower end of the range of estimates found in the literature.
"Estimation and Inference of Average Treatment Effect in Percentage Points under Heterogeneity" (arXiv link)
In semi-log regression models with heterogeneous treatment effects, the average treatment effect (ATE) in log points and its exponential transformation minus one underestimate the ATE in percentage points. I propose new estimation and inference methods for the ATE in percentage points, with inference utilizing the Fenton-Wilkinson approximation. These methods are particularly relevant for staggered difference-in-differences designs, where treatment effects often vary across groups and periods. I prove the methods' large-sample properties and demonstrate their finite-sample performance through simulations, revealing substantial discrepancies between conventional and proposed measures. Two empirical applications further underscore the practical importance of these methods."Mostly Pointless Spatial Econometrics or Not?” (with Guido Kuersteiner and Ingmar Prucha)